The following article is provided by Marlena Lee, Vice President at Dimensional Fund Advisors (DFA), on the value of advice when working with investment and retirement models.
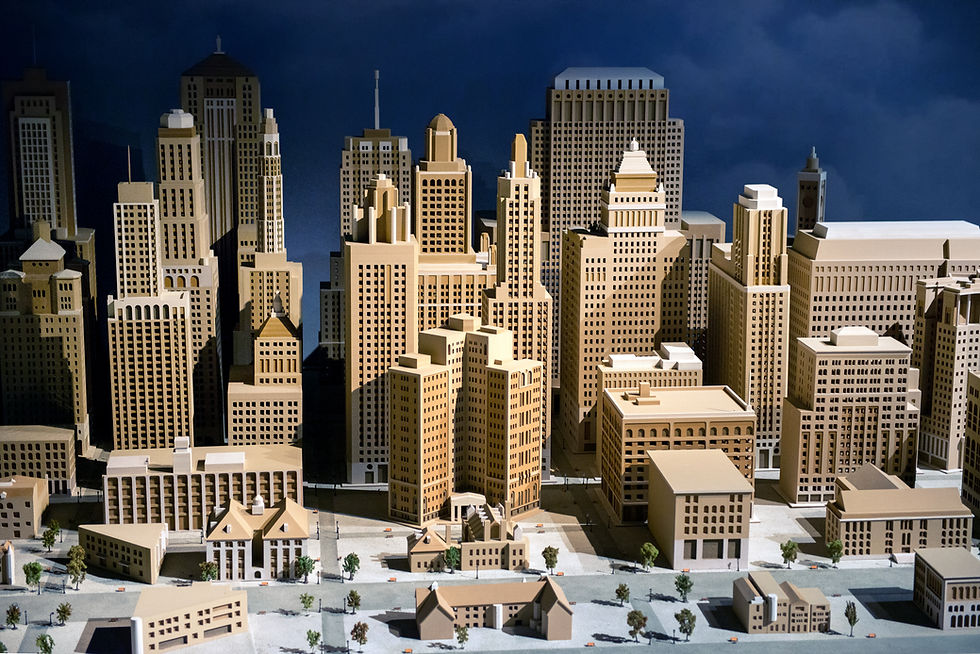
Models are approximations of the world. They are simplifications of reality. Models can be useful for gaining insights that help us make good decisions. But they can also be dangerous if someone is overconfident and does not understand the limitations of models. Bringing financial research to life requires the expertise to distinguish what is worth pursuing vs. what opens the door to unnecessary risks. This column highlights (1) the tradeoffs that we believe must be considered when evaluating models and (2) research ideas in order to build robust portfolios for clients.
A well-known financial model is Harry Markowitz’s relation between expected return and volatility. It is a simple model that gives insights about the importance of diversification. However, when using this framework to inform asset allocation decisions, placing a high degree of faith in inherently imprecise inputs can expose the user to extreme outcomes. Bad financial outcomes have been blamed on modern portfolio theory when actually the fault should be placed on the misuse of that theory.
A researcher working on asset pricing might propose a model or hypothesis about the drivers of expected returns. A good model is one that is testable with data and that yields useful insights about financial markets. However, no model is a perfect representation of reality. Market prices depend on much richer an information set than can be captured by any model. This means that instead of asking “Is this model true or false?” (to which the answer is always false), it is better to ask “How does this model help me better understand markets?” and “In what ways can the model go wrong?”
Type I vs. Type II Error
For example, consider the decision facing the Food and Drug Administration when they assess a new drug. If they do not approve the drug, they give up the potential benefit that the drug may be able to help people. On the other hand, if they approve the drug, the risk is that the drug may not have sufficient health benefits that offset the risk of dangerous side effects.
This tradeoff can be framed as a balance between type I and type II error. Type I error, or a false positive, occurs if researchers approve a drug that is not beneficial or has high risk of harmful side effects. Type II error, or a false negative, occurs if a beneficial drug fails to get approval. If you minimize one error, the chance of the other becomes larger.
Different people may look at the same data and come to different conclusions depending on how much weight they give to type I vs. type II error. Uncertainty about the outcomes may also lead different people to different conclusions. For example, some patients might be happy to try an experimental drug without a proven track record, while others may be less willing.
Investment Applications
When evaluating asset pricing research, we need to evaluate a similar tradeoff. What is the potential benefit a new premium or enhancement can bring to the portfolio? What are the potential costs, and how might we reduce those costs through implementation? How much uncertainty is there around these estimates?
This tradeoff can be reframed in terms of type I and type II error. Type I error occurs if something gets added to the portfolio but does not have an expected net benefit. It is the risk of implementing a bad idea. Type II error occurs if a research idea is not implemented but would have a net benefit to the portfolio. It is the risk of not implementing a good idea. Type I error can be minimized by never making portfolio enhancements. This might describe a traditional index fund approach. Type II error is minimized by having a low bar for the implementation of new ideas. This might describe a quant approach that uses many signals with the hope that there are enough good signals to offset the bad.
If you have to pick one type of error to minimize, the evidence from many performance studies suggests that it should be to minimize the risk of implementing a bad idea. It is difficult for active managers to beat benchmark indices, suggesting that performance-enhancing ideas are not that easy to come by.1 However, the question should not be which error to minimize. We believe more robust portfolios and better investment outcomes can result from balancing both types of errors.
Type I: Defending against Bad Ideas and Unnecessarily High Costs
There are a number of ways to reduce the risk of implementing a bad idea. One way is to defend against data mining by considering whether a premium is sensible and backed by robust empirical evidence. However, even good economic rationale combined with solid empirical research cannot completely eliminate uncertainty. We may have confidence a premium is positive, but expected returns are still only estimates and never guarantee a particular outcome.
Even with a good idea, type I error can result from poor implementation. This is why it is important to make sure the costs of pursuing the idea are low. Costs might come in the form of trading costs, which is why we look at whether an idea can be implemented with low turnover. Costs can also come in the form of reduced diversification, which is why we examine whether an idea can be implemented in portfolios that are well diversified across issuers, sectors, and countries, when relevant.
Pushing too hard on a model or idea can magnify the risk of type I error and increase the probability of catastrophic outcomes. An investor can have high conviction in the size premium but may still not want to have a portfolio of the 10 smallest companies. The momentum premium is robust in historical simulations, but do you have enough conviction that it will be high enough in the future to warrant high turnover? Quant managers, in particular those using multiple (and often times frequently changing) signals, have higher probability of maximizing false positives due to uncertainty about their models and their inputs.
Dimensions of expected returns are premiums in which we have the highest level of confidence because they are sensible, persistent, pervasive, robust, and cost-effective to pursue in well-diversified portfolios. Other examples of financial research might not rise to the level of a dimension but may still be considered as a portfolio enhancement if costs are low. For example, using momentum as a reason to delay trades does not increase the level of turnover. The cost per unit of turnover should not increase because traders can be even more patient when trading. And if momentum disappears in the future, the portfolios will still have potential expected outperformance over benchmarks because they target size, value, and profitability premiums.
What Are We Missing (Type II Error, False Negatives)?
Type II error occurs when we pass on research that may have benefited the portfolio. But how large are these forgone benefits? Given that the majority of active managers fail to beat passive benchmarks, it seems reasonable to conclude that value-enhancing ideas are hard to come by. We believe this suggests that one should be more cautious about implementing a bad idea than worrying about missing out on a good idea.
There is also reason to believe type II error is small, relative to type I error, for Dimensional strategies that already incorporate several value adds. Market, size, relative price, and profitability dimensions already explain a substantial portion of differences in average returns. Exclusions such as small low profitability and enhancements such as the momentum screens further improve expected returns. There is diminishing marginal benefit associated with each addition to a portfolio, and the probability that the benefits from additions will overcome costs becomes smaller. We are committed to continually investigating new enhancements to the strategies, and we work hard to extract every basis point of value add and cost savings for our clients. But we must also be vigilant against adding potentially detrimental changes to the portfolio. This is why we are skeptical when evaluating new research. Given the quality of our current strategies, we keep a high bar to approve any perceived enhancements since the marginal benefit is likely small, while the probability of harmful consequences due to type I errors likely increases.
Understanding How Risk Is Managed Allows for Trust and Long-Term Investor Discipline
In order for investors to stick with their investment choices over the long term and through varying market conditions, they must have confidence that their managers (or advisors) are making wise investment decisions. However, an investor’s ability to evaluate a manager depends greatly on the transparency of the investment process. If it is difficult for investors to understand what investment decisions are being made and why, investors will only be able to evaluate on past performance. We believe investors attracted by past performance alone are likely to redeem when returns are poor.
Investors who are unwilling to trust the decisions of an investment manager might instead put their trust in the market. One way to do this is by holding market cap-weighted portfolios, which are often approximated with investments in index funds or ETFs. The goal of an index fund manager is to minimize tracking error relative to a specified benchmark, leaving the manager with close to zero investment decision-making authority. By outsourcing the portfolio construction, an index fund manager can achieve high levels of transparency while requiring little to no trust on the part of the investor. When investment returns are poor, investors can blame it on the market, rather than on a bad call by the manager.
Trust in the market can be implemented in a less rigid way than an index fund. Dimensional’s investment process relies on current market prices to identify differences in expected returns across securities. We apply discretion when we weigh the risk of type I and type II error in deciding what premiums to pursue. We must apply expertise when designing portfolios to target the premiums we determine are worthwhile and when managing the tradeoffs that arise as prices move every day—all done with an eye toward reducing execution costs.
Our clients place trust in our ability to exercise good judgment in each of these steps, and we work hard to earn and retain that trust by making decisions based on solid economic rationale and robust empirical research. To place trust in Dimensional is to place trust in market prices and in the collective wisdom of thousands of market participants. It is to place trust in robust research that is well accepted throughout the academic community. Unlike the blind trust that is required of an opaque stock picker or quant manager, we earn clients’ trust through reason, research, and 35 years of experience. We believe this trust helps clients be better equipped to weather periods of underperformance and is the key for fostering the discipline needed to seek better investment outcomes.
Glossary:
Basis Point (bp): One basis point equals 0.01%. Data Mining: The process of analyzing data for trends or patterns that exist independent of a sensible causal relationship and/or in order to fit a pre-established conclusion. False Negative: A test result that incorrectly indicates a particular condition or attribute is absent. False Positive: A test result that incorrectly indicates that a particular condition or attribute is present. Momentum: The empirically observed tendency of securities with recent relative outperformance to continue to outperform on a relative basis over short periods of time (three to 12 months) and of securities with recent relative underperformance to continue to underperform on a relative basis over short periods of time. Premium: A return difference between two assets or portfolios. Profitability Premium: The return difference between stocks of companies with high profitability over those with low profitability. Quantitative Approach: An investment approach that selects investments based on mathematical measurements and statistical modeling. Signal: A pattern or indicator in a data series that is perceived to be meaningful. Size Premium or Small Cap Premium: The return difference between small capitalization stocks and large capitalization stocks. Tracking Error: A measure used to quantify how closely a portfolio follows an index or benchmark, often defined as the standard deviation of the difference between the portfolio and index returns. Value Premium: The return difference between stocks with low relative prices (value) and stocks with high relative prices (growth).
1.For example, see Fama and French (2010), “Luck vs. Skill in the Cross Section of Mutual Fund Returns.”